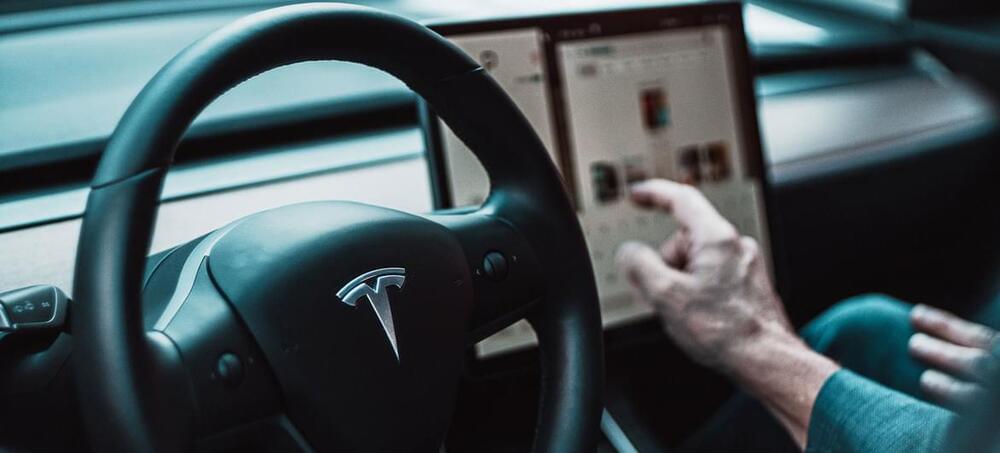
The Sustainable Development Goals (SDGs) include a call for action to halve the annual rate of road deaths globally and ensure access to safe, affordable, and sustainable transport for everyone by 2030.
According to the newly launched initiative, faster progress on AI is vital to make this happen, especially in low and middle-income countries, where the most lives are lost on the roads each year.
According to the World Health Organization (WHO), approximately 1.3 million people die annually as a result of road traffic crashes. Between 20 and 50 million more suffer non-fatal injuries, with many incurring a disability.
Full Story:
A woman rushes across a busy road in Brazil., by PAHO
AI can help in different ways, including better collection and analysis of crash data, enhancing road infrastructure, increasing the efficiency of post-crash response, and inspiring innovation in the regulatory frameworks.
This approach requires equitable access to data and the ethical use of algorithms, which many countries currently lack, leaving them unable to identify road safety solutions.