Reinforcement learning (RL) is a powerful type of AI technology that can learn strategies to optimally control large, complex systems.
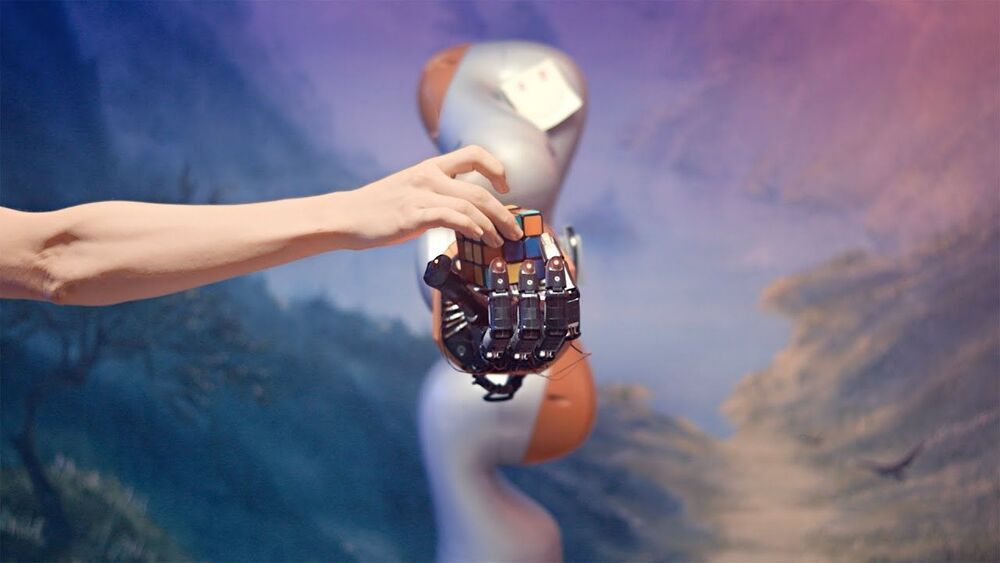
Reinforcement learning (RL) is a powerful type of AI technology that can learn strategies to optimally control large, complex systems.
Deep neural networks exploit statistical regularities in data to carry out prediction or classification tasks. This makes them very good at handling computer vision tasks such as detecting objects. But reliance on statistical patterns also makes neural networks sensitive to adversarial examples.
An adversarial example is an image that has been subtly modified to cause a deep learning model to misclassify it. This usually happens by adding a layer of noise to a normal image. Each noise pixel changes the numerical values of the image very slightly, enough to be imperceptible to the human eye. But when added together, the noise values disrupt the statistical patterns of the image, which then causes a neural network to mistake it for something else.
The military’s primary advanced research shop wants to be a leader in the “third wave” of artificial intelligence and is looking at new methods of visually tracking objects using significantly less power while producing results that are 10-times more accurate.
The Defense Advanced Research Projects Agency, or DARPA, has been instrumental in many of the most important breakthroughs in modern technology—from the first computer networks to early AI research.
“DARPA-funded R&D enabled some of the first successes in AI, such as expert systems and search, and more recently has advanced machine learning algorithms and hardware,” according to a notice for an upcoming opportunity.
A team of researchers at the University of Georgia has created a backpack equipped with AI gear aimed at replacing guide dogs and canes for the blind. Intel has published a News Byte describing the new technology on their Newsroom page.
Technology to help blind people get around in public has been improving in recent years, thanks mostly to smartphone apps. But such apps, the team notes, are not sufficient given the technology available. To make a better assistance system, the group designed an AI system that could be placed in a backpack and worn by a blind person to give them much better clues about their environment.
The backpack holds a smart AI system running on a laptop, and is fitted with OAK-D cameras (which, in addition to providing obstacle information, can also provide depth information) hidden in a vest and also in a waist pack. The cameras run Intel’s Movidius VPU and are programmed using the OpenVINO toolkit. The waist pack also holds batteries for the system. The AI system was trained to recognize objects a sighted pedestrian would see when walking around in a town or city, such as cars, bicycles, other pedestrians or even overhanging tree limbs.
A “self-portrait” by humanoid robot Sophia, who “interpreted” a depiction of her own face, has sold at auction for over $688000.
A hand-painted “self-portrait” by the world-famous humanoid robot, Sophia, has sold at auction for over $688000.
The work, which saw Sophia “interpret” a depiction of her own face, was offered as a non-fungible token, or NFT, an encrypted digital signature that has revolutionized the art market in recent months.
Titled “Sophia Instantiation,” the image was created in collaboration with Andrea Bonaceto, an artist and partner at blockchain investment firm Eterna Capital. Bonaceto began the process by producing a brightly colored portrait of Sophia, which was processed by the robot’s neural networks. Sophia then painted an interpretation of the image.
Artificial microswimmers that can replicate the complex behavior of active matter are often designed to mimic the self-propulsion of microscopic living organisms. However, compared with their living counterparts, artificial microswimmers have a limited ability to adapt to environmental signals or to retain a physical memory to yield optimized emergent behavior. Different from macroscopic living systems and robots, both microscopic living organisms and artificial microswimmers are subject to Brownian motion, which randomizes their position and propulsion direction. Here, we combine real-world artificial active particles with machine learning algorithms to explore their adaptive behavior in a noisy environment with reinforcement learning. We use a real-time control of self-thermophoretic active particles to demonstrate the solution of a simple standard navigation problem under the inevitable influence of Brownian motion at these length scales. We show that, with external control, collective learning is possible. Concerning the learning under noise, we find that noise decreases the learning speed, modifies the optimal behavior, and also increases the strength of the decisions made. As a consequence of time delay in the feedback loop controlling the particles, an optimum velocity, reminiscent of optimal run-and-tumble times of bacteria, is found for the system, which is conjectured to be a universal property of systems exhibiting delayed response in a noisy environment.
Living organisms adapt their behavior according to their environment to achieve a particular goal. Information about the state of the environment is sensed, processed, and encoded in biochemical processes in the organism to provide appropriate actions or properties. These learning or adaptive processes occur within the lifetime of a generation, over multiple generations, or over evolutionarily relevant time scales. They lead to specific behaviors of individuals and collectives. Swarms of fish or flocks of birds have developed collective strategies adapted to the existence of predators (1), and collective hunting may represent a more efficient foraging tactic (2). Birds learn how to use convective air flows (3). Sperm have evolved complex swimming patterns to explore chemical gradients in chemotaxis (4), and bacteria express specific shapes to follow gravity (5).
Inspired by these optimization processes, learning strategies that reduce the complexity of the physical and chemical processes in living matter to a mathematical procedure have been developed. Many of these learning strategies have been implemented into robotic systems (7–9). One particular framework is reinforcement learning (RL), in which an agent gains experience by interacting with its environment (10). The value of this experience relates to rewards (or penalties) connected to the states that the agent can occupy. The learning process then maximizes the cumulative reward for a chain of actions to obtain the so-called policy. This policy advises the agent which action to take. Recent computational studies, for example, reveal that RL can provide optimal strategies for the navigation of active particles through flows (11–13), the swarming of robots (14–16), the soaring of birds , or the development of collective motion (17).
“Genius Makers” and “Futureproof,” both by experienced technology reporters now at The New York Times, are part of a rapidly growing literature attempting to make sense of the A.I. hurricane we are living through. These are very different kinds of books — Cade Metz’s is mainly reportorial, about how we got here; Kevin Roose’s is a casual-toned but carefully constructed set of guidelines about where individuals and societies should go next. But each valuably suggests a framework for the right questions to ask now about A.I. and its use.
Two new books — “Genius Makers,” by Cade Metz, and “Futureproof,” by Kevin Roose — examine how artificial intelligence will change humanity.