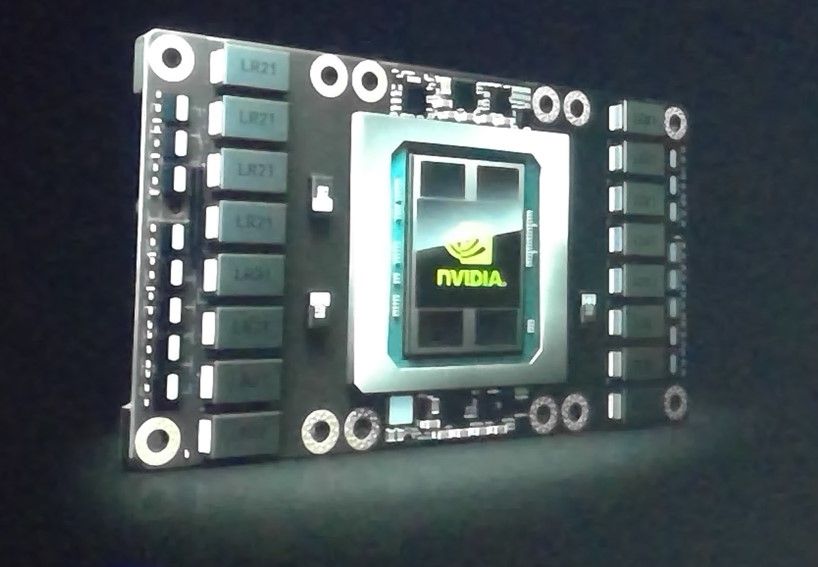
At a time when PCs have become rather boring and the market has stagnated, the Graphics Processing Unit (GPU) has become more interesting and not for what it has traditionally done (graphical user interface), but for what it can do going forward. GPUs are a key enabler for the PC and workstation market, both for enthusiast seeking to increase graphics performance for games and developers and designers looking to create realistic new videos and images. However, the traditional PC market has been in decline for several years as consumer shift to mobile computing solutions like smartphones. At the same time, the industry has been working to expand the use of GPUs as a computing accelerator because of the massive parallel compute capabilities, often providing the horsepower for top supercomputers. NVIDIA has been a pioneer in this GPU compute market with its CUDA platform, enabling leading researchers to perform leading edge research and continue to develop new uses for GPU acceleration.
Now, the industry is looking to leverage over 40 years of GPU history and innovation to create more advanced computer intelligence. Through the use of sensors, increased connectivity, and new learning technique, researchers can enable artificial intelligence (AI) applications for everything from autonomous vehicles to scientific research. This, however, requires unprecedented levels of computing power, something the NVIDIA is driven to provide. At the GPU Technology Conference (GTC) in San Jose, California, NVIDIA just announced a new GPU platform that takes computing to the extreme. NVIDIA introduced the Telsa P100 platform. NVIDIA CEO Jen-Hsun Huang described the Tesla P100 as the first GPU designed for hyperscale datacenter applications. It features NVIDIA’s new Pascal GPU architecture, the latest memory and semiconductor process, and packaging technology – all to create the densest compute platform to date.
Read more