Abstract: Advances in high speed imaging techniques have opened new possibilities for capturing ultrafast phenomena such as light propagation in air or through media. Capturing light-in-flight in 3-dimensional xyt-space has been reported based on various types of imaging systems, whereas reconstruction of light-in-flight information in the fourth dimension z has been a challenge. We demonstrate the first 4-dimensional light-in-flight imaging based on the observation of a superluminal motion captured by a new time-gated megapixel single-photon avalanche diode camera. A high resolution light-in-flight video is generated with no laser scanning, camera translation, interpolation, nor dark noise subtraction. A machine learning technique is applied to analyze the measured spatio-temporal data set. A theoretical formula is introduced to perform least-square regression, and extra-dimensional information is recovered without prior knowledge. The algorithm relies on the mathematical formulation equivalent to the superluminal motion in astrophysics, which is scaled by a factor of a quadrillionth. The reconstructed light-in-flight trajectory shows a good agreement with the actual geometry of the light path. Our approach could potentially provide novel functionalities to high speed imaging applications such as non-line-of-sight imaging and time-resolved optical tomography.
Category: information science

How to make AI trustworthy
One of the biggest impediments to adoption of new technologies is trust in AI.
Now, a new tool developed by USC Viterbi Engineering researchers generates automatic indicators if data and predictions generated by AI algorithms are trustworthy. Their research paper, “There Is Hope After All: Quantifying Opinion and Trustworthiness in Neural Networks” by Mingxi Cheng, Shahin Nazarian and Paul Bogdan of the USC Cyber Physical Systems Group, was featured in Frontiers in Artificial Intelligence.
Neural networks are a type of artificial intelligence that are modeled after the brain and generate predictions. But can the predictions these neural networks generate be trusted? One of the key barriers to adoption of self-driving cars is that the vehicles need to act as independent decision-makers on auto-pilot and quickly decipher and recognize objects on the road—whether an object is a speed bump, an inanimate object, a pet or a child—and make decisions on how to act if another vehicle is swerving towards it.

Scientists use reinforcement learning to train quantum algorithm
Recent advancements in quantum computing have driven the scientific community’s quest to solve a certain class of complex problems for which quantum computers would be better suited than traditional supercomputers. To improve the efficiency with which quantum computers can solve these problems, scientists are investigating the use of artificial intelligence approaches.
In a new study, scientists at the U.S. Department of Energy’s (DOE) Argonne National Laboratory have developed a new algorithm based on reinforcement learning to find the optimal parameters for the Quantum Approximate Optimization Algorithm (QAOA), which allows a quantum computer to solve certain combinatorial problems such as those that arise in materials design, chemistry and wireless communications.
“Combinatorial optimization problems are those for which the solution space gets exponentially larger as you expand the number of decision variables,” said Argonne computer scientist Prasanna Balaprakash. “In one traditional example, you can find the shortest route for a salesman who needs to visit a few cities once by enumerating all possible routes, but given a couple thousand cities, the number of possible routes far exceeds the number of stars in the universe; even the fastest supercomputers cannot find the shortest route in a reasonable time.”
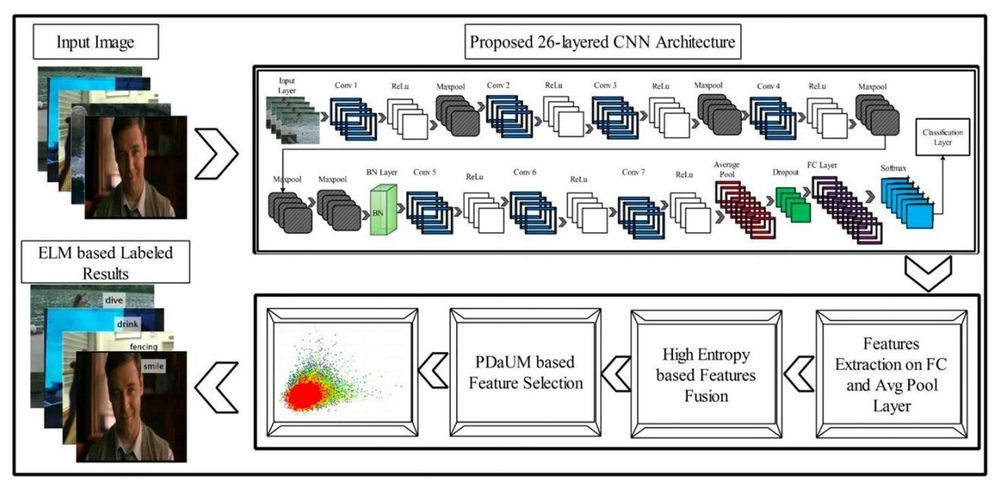
A 26-layer convolutional neural network for human action recognition
Deep learning algorithms, such as convolutional neural networks (CNNs), have achieved remarkable results on a variety of tasks, including those that involve recognizing specific people or objects in images. A task that computer scientists have often tried to tackle using deep learning is vision-based human action recognition (HAR), which specifically entails recognizing the actions of humans who have been captured in images or videos.
Researchers at HITEC University and Foundation University Islamabad in Pakistan, Sejong University and Chung-Ang University in South Korea, University of Leicester in the UK, and Prince Sultan University in Saudi Arabia have recently developed a new CNN for recognizing human actions in videos. This CNN, presented in a paper published in Springer Link’s Multimedia Tools and Applications journal, was trained to differentiate between several different human actions, including boxing, clapping, waving, jogging, running and walking.
“We designed a new 26-layered convolutional neural network (CNN) architecture for accurate complex action recognition,” the researchers wrote in their paper. “The features are extracted from the global average pooling layer and fully connected (FC) layer and fused by a proposed high entropy-based approach.”
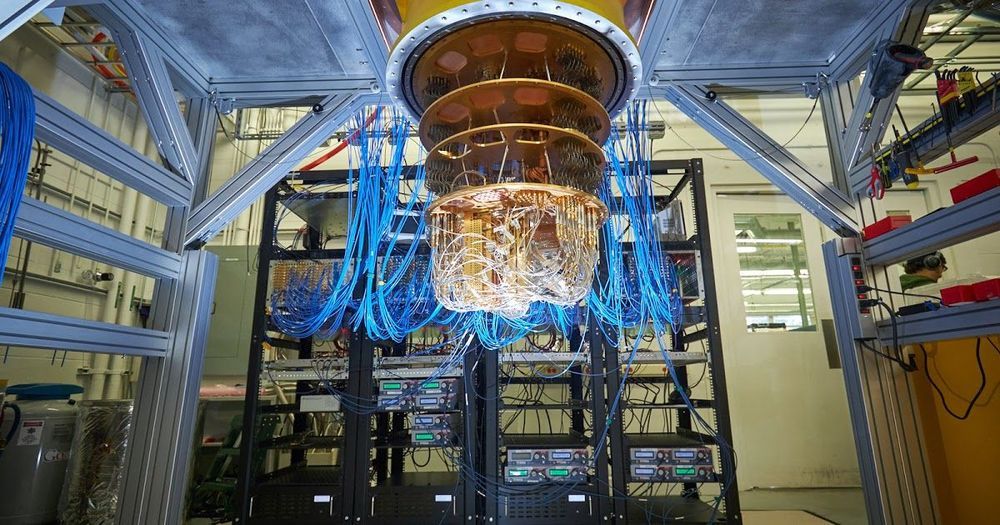
Scaling Up Fundamental Quantum Chemistry Simulations on Quantum Hardware
Accurate computational prediction of chemical processes from the quantum mechanical laws that govern them is a tool that can unlock new frontiers in chemistry, improving a wide variety of industries. Unfortunately, the exact solution of quantum chemical equations for all but the smallest systems remains out of reach for modern classical computers, due to the exponential scaling in the number and statistics of quantum variables. However, by using a quantum computer, which by its very nature takes advantage of unique quantum mechanical properties to handle calculations intractable to its classical counterpart, simulations of complex chemical processes can be achieved. While today’s quantum computers are powerful enough for a clear computational advantage at some tasks, it is an open question whether such devices can be used to accelerate our current quantum chemistry simulation techniques.
In “Hartree-Fock on a Superconducting Qubit Quantum Computer”, appearing today in Science, the Google AI Quantum team explores this complex question by performing the largest chemical simulation performed on a quantum computer to date. In our experiment, we used a noise-robust variational quantum eigensolver (VQE) to directly simulate a chemical mechanism via a quantum algorithm. Though the calculation focused on the Hartree-Fock approximation of a real chemical system, it was twice as large as previous chemistry calculations on a quantum computer, and contained ten times as many quantum gate operations. Importantly, we validate that algorithms being developed for currently available quantum computers can achieve the precision required for experimental predictions, revealing pathways towards realistic simulations of quantum chemical systems.

Can you make AI fairer than a judge? Play our courtroom algorithm game
As a child, you develop a sense of what “fairness” means. It’s a concept that you learn early on as you come to terms with the world around you. Something either feels fair or it doesn’t.
But increasingly, algorithms have begun to arbitrate fairness for us. They decide who sees housing ads, who gets hired or fired, and even who gets sent to jail. Consequently, the people who create them—software engineers—are being asked to articulate what it means to be fair in their code. This is why regulators around the world are now grappling with a question: How can you mathematically quantify fairness?
This story attempts to offer an answer. And to do so, we need your help. We’re going to walk through a real algorithm, one used to decide who gets sent to jail, and ask you to tweak its various parameters to make its outcomes more fair. (Don’t worry—this won’t involve looking at code!)

Artificial Intelligence Is Getting Insanely Good at Removing Shadows From Photographs of Faces
While Photoshop can do a pretty good job at removing shadows from faces, there’s a fair amount of legwork involved. One scientist has shown that neural networks and artificial intelligence can produce some very impressive results, suggesting that it will soon be a part of how we edit our photos.
Károly Zsolnai-Fehér of Two Minute Papers and the Institute of Computer Graphics and Algorithms, Vienna University of Technology, Austria, just released a video demonstrating how he has taught a neural network using large data sets to recognize and eliminate shadows from a face in a photograph. As detailed in the video, the neural network was taught by giving it photographs of faces to which shadows had been added artificially.
Given its effectiveness and the quality of the results, it seems only a matter of time before smartphones give you the option to remove shadows. In theory, you might even be able to switch on shadow removal while taking the photograph.
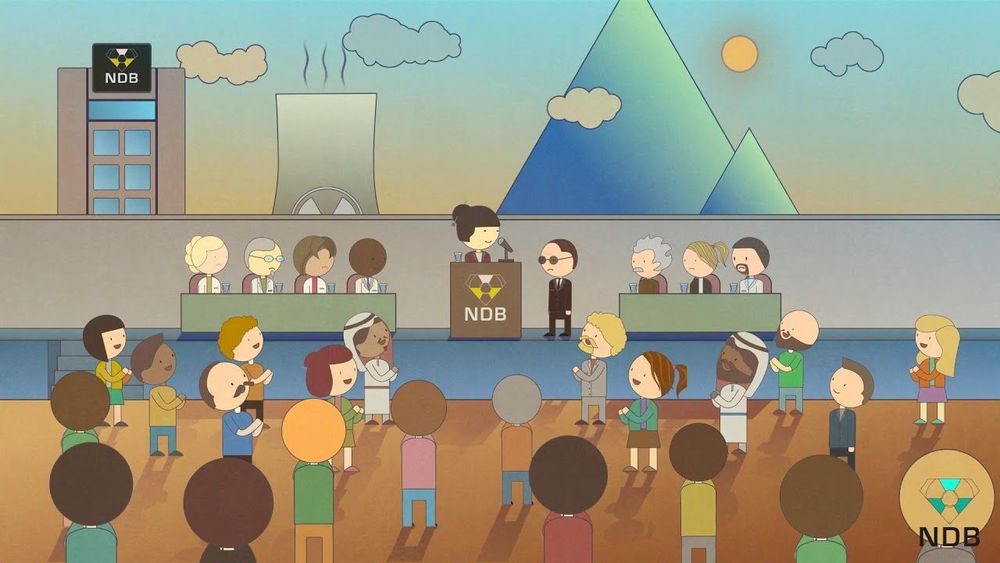
Nano-diamond self-charging batteries could disrupt energy as we know it
https://youtube.com/watch?v=ksMXbhftBbM
California company NDB says its nano-diamond batteries will absolutely upend the energy equation, acting like tiny nuclear generators. They will blow any energy density comparison out of the water, lasting anywhere from a decade to 28,000 years without ever needing a charge. They will offer higher power density than lithium-ion. They will be nigh-on indestructible and totally safe in an electric car crash. And in some applications, like electric cars, they stand to be considerably cheaper than current lithium-ion packs despite their huge advantages.
The heart of each cell is a small piece of recycled nuclear waste. NDB uses graphite nuclear reactor parts that have absorbed radiation from nuclear fuel rods and have themselves become radioactive. Untreated, it’s high-grade nuclear waste: dangerous, difficult and expensive to store, with a very long half-life.
This graphite is rich in the carbon-14 radioisotope, which undergoes beta decay into nitrogen, releasing an anti-neutrino and a beta decay electron in the process. NDB takes this graphite, purifies it and uses it to create tiny carbon-14 diamonds. The diamond structure acts as a semiconductor and heat sink, collecting the charge and transporting it out. Completely encasing the radioactive carbon-14 diamond is a layer of cheap, non-radioactive, lab-created carbon-12 diamond, which contains the energetic particles, prevents radiation leaks and acts as a super-hard protective and tamper-proof layer.

The term ‘ethical AI’ is finally starting to mean something
Since OpenAI first described its new AI language-generating system called GPT-3 in May, hundreds of media outlets (including MIT Technology Review) have written about the system and its capabilities. Twitter has been abuzz about its power and potential. The New York Times published an op-ed about it. Later this year, OpenAI will begin charging companies for access to GPT-3, hoping that its system can soon power a wide variety of AI products and services.
Earlier this year, the independent research organisation of which I am the Director, London-based Ada Lovelace Institute, hosted a panel at the world’s largest AI conference, CogX, called The Ethics Panel to End All Ethics Panels. The title referenced both a tongue-in-cheek effort at self-promotion, and a very real need to put to bed the seemingly endless offering of panels, think-pieces, and government reports preoccupied with ruminating on the abstract ethical questions posed by AI and new data-driven technologies. We had grown impatient with conceptual debates and high-level principles.
And we were not alone. 2020 has seen the emergence of a new wave of ethical AI – one focused on the tough questions of power, equity, and justice that underpin emerging technologies, and directed at bringing about actionable change. It supersedes the two waves that came before it: the first wave, defined by principles and dominated by philosophers, and the second wave, led by computer scientists and geared towards technical fixes. Third-wave ethical AI has seen a Dutch Court shut down an algorithmic fraud detection system, students in the UK take to the streets to protest against algorithmically-decided exam results, and US companies voluntarily restrict their sales of facial recognition technology. It is taking us beyond the principled and the technical, to practical mechanisms for rectifying power imbalances and achieving individual and societal justice.
Between 2016 and 2019, 74 sets of ethical principles or guidelines for AI were published. This was the first wave of ethical AI, in which we had just begun to understand the potential risks and threats of rapidly advancing machine learning and AI capabilities and were casting around for ways to contain them. In 2016, AlphaGo had just beaten Lee Sedol, promoting serious consideration of the likelihood that general AI was within reach. And algorithmically-curated chaos on the world’s duopolistic platforms, Google and Facebook, had surrounded the two major political earthquakes of the year – Brexit, and Trump’s election.
