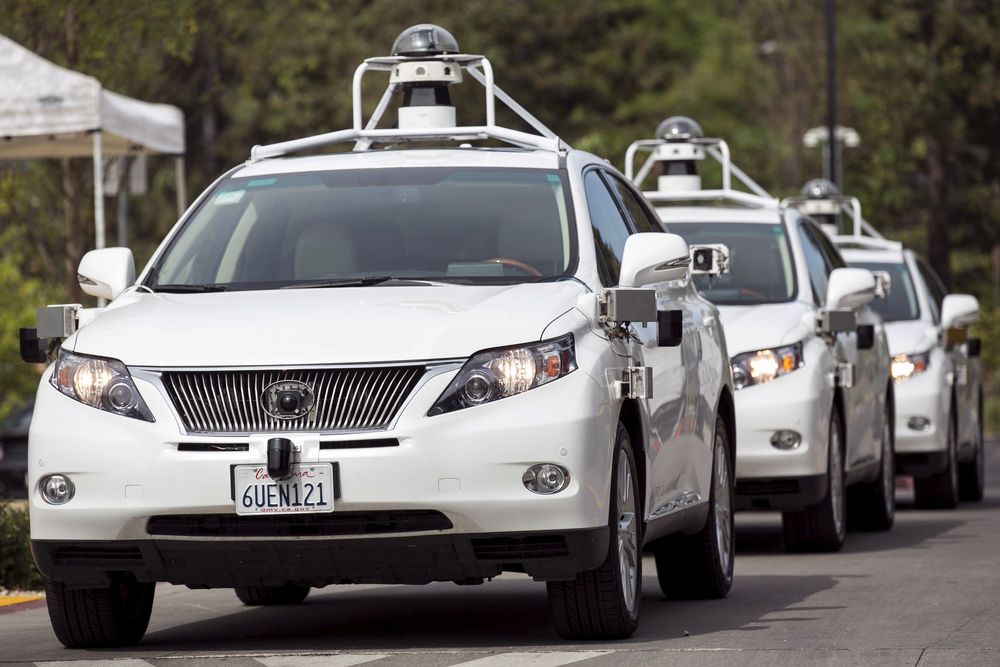
Say something Eric Klien.
Given the increasing proliferation of AI, I recently carried out a systematic review of AI-driven regulatory gaps. My review sampled the academic literature on AI in the hard and social sciences and found fifty existing or future regulatory gaps caused by this technology’s applications and methods in the United States. Drawing on an adapted version of Lyria Bennett-Moses’s framework, I then characterized each regulatory gap according to one of four categories: novelty, obsolescence, targeting, and uncertainty.
Significantly, of the regulatory gaps identified, only 12 percent represent novel challenges that compel government action through the creation or adaptation of regulation. By contrast, another 20 percent of the gaps are cases in which AI has made or will make regulations obsolete. A quarter of the gaps are problems of targeting, in which regulations are either inappropriately applied to AI or miss cases in which they should be applied. The largest group of regulatory gaps are ones of uncertainty in which a new technology is difficult to classify, causing a lack of clarity about the application of existing regulations.
Novelty. In cases of novel regulatory gaps, a technology creates behavior that requires bespoke government action. Of the identified cases, 12 percent are novel. This includes, for example, the Food and Drug Administration’s (FDA) standard for certifying the safety of high-risk medical devices which is applicable to healthcare algorithms, also called black-box medicine.