A revolutionary new class of amphibious vehicle will transform the search for lost vessels on the ocean floor, says marine archaeologist Dr Robert Ballard.
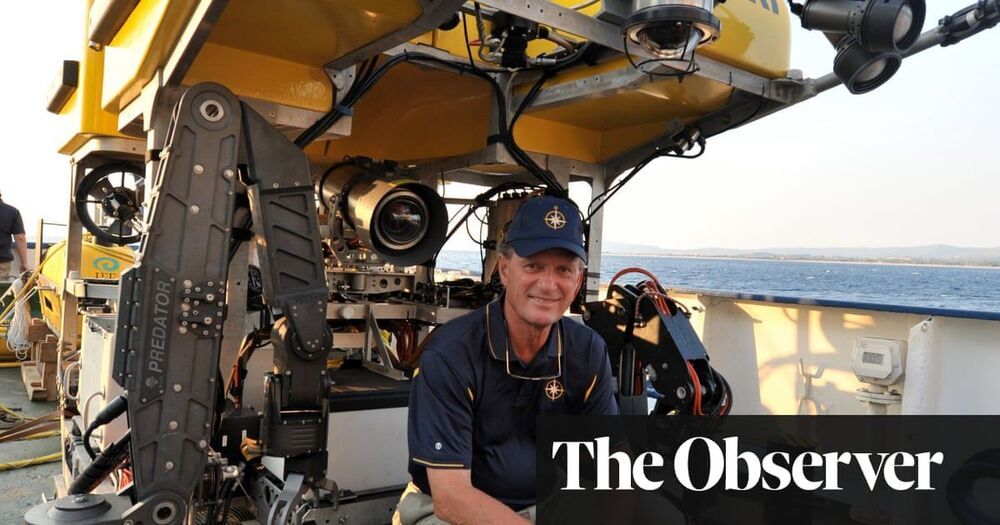
Researchers have demonstrated how to keep a network of nanowires in a state that’s right on what’s known as the edge of chaos – an achievement that could be used to produce artificial intelligence (AI) that acts much like the human brain does.
The team used varying levels of electricity on a nanowire simulation, finding a balance when the electric signal was too low when the signal was too high. If the signal was too low, the network’s outputs weren’t complex enough to be useful; if the signal was too high, the outputs were a mess and also useless.
“We found that if you push the signal too slowly the network just does the same thing over and over without learning and developing. If we pushed it too hard and fast, the network becomes erratic and unpredictable,” says physicist Joel Hochstetter from the University of Sydney and the study’s lead author.
“What’s so exciting about this result is that it suggests that these types of nanowire networks can be tuned into regimes with diverse, brain-like collective dynamics, which can be leveraged to optimize information processing,” said Zdenka Kuncic from the University of Sydney in a press release.
Today’s deep neural networks already mimic one aspect of the brain: its highly interconnected network of neurons. But artificial neurons behave very differently than biological ones, as they only carry out computations. In the brain, neurons are also able to remember their previous activity, which then influences their future behavior.
This in-built memory is a crucial aspect of how the brain processes information, and a major strand in neuromorphic engineering focuses on trying to recreate this functionality. This has resulted in a wide range of designs for so-called “memristors”: electrical components whose response depends on the previous signals they have been exposed to.
Voice assistants are a very controversial technological implementation, as some believe they are too intrusive with users’ privacy and do not provide significantly useful features compared to using a conventional computer or smartphone. Its use becomes increasingly questionable considering the constant reports of information collection. The most recent of these reports points out that Google Assistant technology can listen to users’ conversations even without being invoked.
The company had already admitted that it records some of the users’ conversations, though its reports claimed this was impossible if the user didn’t say “OK Google” aloud. However, this new report ensures that it is not necessary for the user to activate the assistant with the renowned voice command.
According to Android Authority researchers, a Google representative admitted that its virtual assistant records audio even when this tool is not used, even recognizing that employees have access to this information. However, Google clarified that this has not been done inadvertently, ensuring that its staff is not listening to users’ conversations. In addition, it was established that Google staff only have access to limited parts of the audio.
Astronomy is all about data. The universe is getting bigger and so too is the amount of information we have about it. But some of the biggest challenges of the next generation of astronomy lie in just how we’re going to study all the data we’re collecting.
To take on these challenges, astronomers are turning to machine learning and artificial intelligence (AI) to build new tools to rapidly search for the next big breakthroughs. Here are four ways AI is helping astronomers.
Physics-informed machine learning might help verify microchips.
Physicists love recreating the world in software. A simulation lets you explore many versions of reality to find patterns or to test possibilities. But if you want one that’s realistic down to individual atoms and electrons, you run out of computing juice pretty quickly.
Machine-learning models can approximate detailed simulations, but often require lots of expensive training data. A new method shows that physicists can lend their expertise to machine-learning algorithms, helping them train on a few small simulations consisting of a few atoms, then predict the behavior of system with hundreds of atoms. In the future, similar techniques might even characterize microchips with billions of atoms, predicting failures before they occur.
The researchers started with simulated units of 16 silicon and germanium atoms, two elements often used to make microchips. They employed high-performance computers to calculate the quantum-mechanical interactions between the atoms’ electrons. Given a certain arrangement of atoms, the simulation generated unit-level characteristics such as its energy bands, the energy levels available to its electrons. But “you realize that there is a big gap between the toy models that we can study using a first-principles approach and realistic structures,” says Sanghamitra Neogi, a physicist at the University of Colorado, Boulder, and the paper’s senior author. Could she and her co-author, Artem Pimachev, bridge the gap using machine learning?
In a new patent from AMD, researchers have highlighted techniques for performing machine learning operations using one or more dedicated ML accelerator chiplets. The resulting device is called an Accelerated Processing Device or APD which can be used for both gaming and data center GPUs via different implementations. The method involves configuring a part of the chiplet memory as a cache while the other as directly accessible memory.
The sub-portion of the former is then used by the machine learning accelerators on the same chiplet to perform machine learning operations. The patent is very open-ended with respect to its uses, indicating possible use in CPUs, GPUs, or other caching devices, but the primary target appears to be GPUs with several thousand SIMD units.
One implementation of the APD is configured to accept commands via both graphics and compute pipelines from the command processor, showing the ability to both render graphics as well as compute-intensive workloads required by convolution networks. The APD contains several SIMDs capable of performing vector operations alongside limited scalar and SP tasks similar to existing AMD GPUs.