“There’s a high-stakes race under way in Silicon Valley to develop software that makes it easy to weave artificial intelligence technology into almost everything, and Google has sprinted into the lead.”
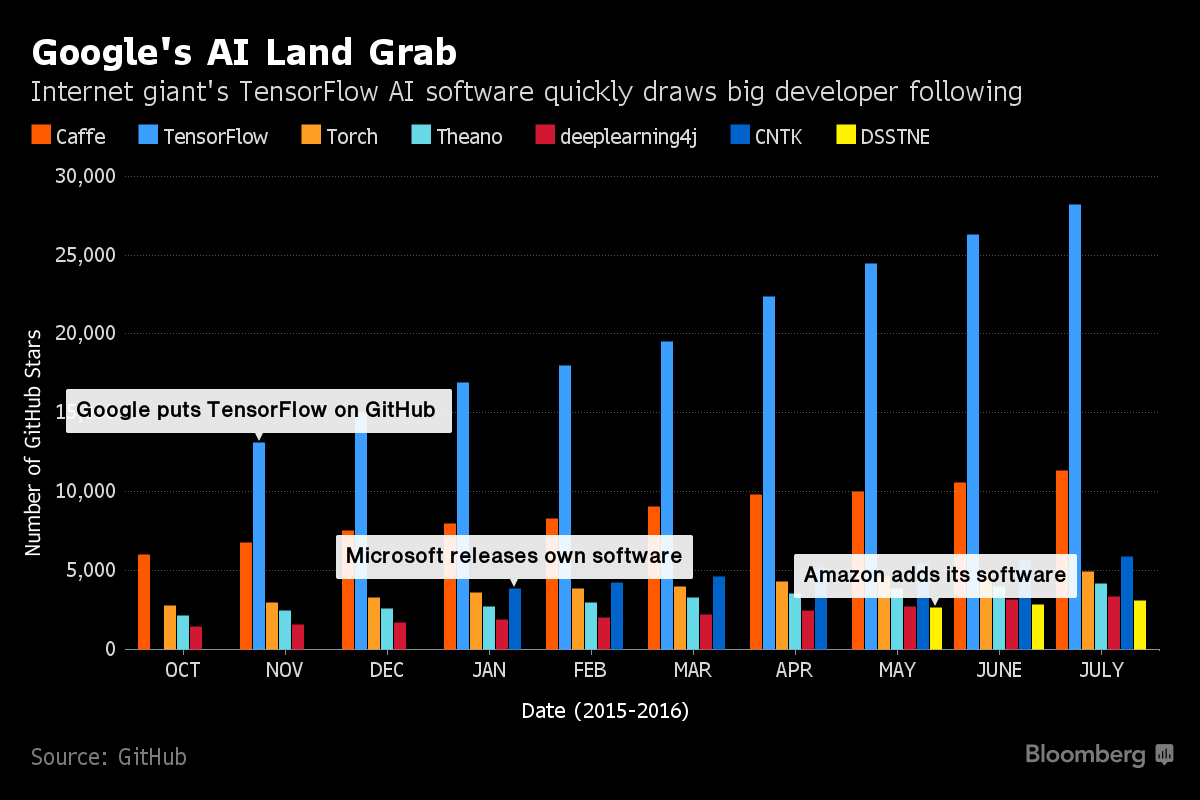
“There’s a high-stakes race under way in Silicon Valley to develop software that makes it easy to weave artificial intelligence technology into almost everything, and Google has sprinted into the lead.”
Podcast with “Andreessen Horowitz Distinguished Visiting Professor of Computer Science … Fei-Fei Li [who publishes under Li Fei-Fei], associate professor at Stanford University.”
Today’s conference emphasizes virtual reality and machine learning.
If you’ve ever seen a “recommended item” on eBay or Amazon that was just what you were looking for (or maybe didn’t know you were looking for), it’s likely the suggestion was powered by a recommendation engine. In a recent interview, Co-founder of machine learning startup Delvv, Inc., Raefer Gabriel, said these applications for recommendation engines and collaborative filtering algorithms are just the beginning of a powerful and broad-reaching technology.
Raefer Gabriel, Delvv, Inc.Gabriel noted that content discovery on services like Netflix, Pandora, and Spotify are most familiar to people because of the way they seem to “speak” to one’s preferences in movies, games, and music. Their relatively narrow focus of entertainment is a common thread that has made them successful as constrained domains. The challenge lies in developing recommendation engines for unbounded domains, like the internet, where there is more or less unlimited information.
“Some of the more unbounded domains, like web content, have struggled a little bit more to make good use of the technology that’s out there. Because there is so much unbounded information, it is hard to represent well, and to match well with other kinds of things people are considering,” Gabriel said. “Most of the collaborative filtering algorithms are built around some kind of matrix factorization technique and they definitely tend to work better if you bound the domain.”
Of all the recommendation engines and collaborative filters on the web, Gabriel cites Amazon as the most ambitious. The eCommerce giant utilizes a number of strategies to make item-to-item recommendations, complementary purchases, user preferences, and more. The key to developing those recommendations is more about the value of the data that Amazon is able to feed into the algorithm initially, hence reaching a critical mass of data on user preferences, which makes it much easier to create recommendations for new users.
“In order to handle those fresh users coming into the system, you need to have some way of modeling what their interest may be based on that first click that you’re able to extract out of them,” Gabriel said. “I think that intersection point between data warehousing and machine learning problems is actually a pretty critical intersection point, because machine learning doesn’t do much without data. So, you definitely need good systems to collect the data, good systems to manage the flow of data, and then good systems to apply models that you’ve built.”
Beyond consumer-oriented uses, Gabriel has seen recommendation engines and collaborative filter systems used in a narrow scope for medical applications and in manufacturing. In healthcare for example, he cited recommendations based on treatment preferences, doctor specialties, and other relevant decision-based suggestions; however, anything you can transform into a “model of relationships between items and item preferences” can map directly onto some form of recommendation engine or collaborative filter.
One of the most important elements that has driven the development of recommendation engines and collaborative filtering algorithms is the Netflix Prize, Gabriel said. The competition, which offered a $1 million prize to anyone who could design an algorithm to improve upon the proprietary Netflix’s recommendation engine, allowed entrants to use pieces of the company’s own user data to develop a better algorithm. The competition spurred a great deal of interest in the potential applications of collaborative filtering and recommendation engines, he said.
In addition, relative ease of access to an abundant amount of cheap memory is another driving force behind the development of recommendation engines. An eCommerce company like Amazon with millions of items needs plenty of memory to store millions of different of pieces of item and correlation data while also storing user data in potentially large blocks.
“You have to think about a lot of matrix data in memory. And it’s a matrix, because you’re looking at relationships between items and other items and, obviously, the problems that get interesting are ones where you have lots and lots of different items,” Gabriel said. “All of the fitting and the data storage does need quite a bit of memory to work with. Cheap and plentiful memory has been very helpful in the development of these things at the commercial scale.”
Looking forward, Gabriel sees recommendation engines and collaborative filtering systems evolving more toward predictive analytics and getting a handle on the unbounded domain of the internet. While those efforts may ultimately be driven by the Google Now platform, he foresees a time when recommendation-driven data will merge with search data to provide search results before you even search for them.
“I think there will be a lot more going on at that intersection between the search and recommendation space over the next couple years. It’s sort of inevitable,” Gabriel said. “You can look ahead to what someone is going to be searching for next, and you can certainly help refine and tune into the right information with less effort.”
While “mind-reading” search engines may still seem a bit like science fiction at present, the capabilities are evolving at a rapid pace, with predictive analytics at the bow.
” “There is going to be a boom for design companies, because there’s going to be so much information people have to work through quickly,” said Diane B. Greene, the head of Google Compute Engine, one of the companies hoping to steer an A.I. boom. “Just teaching companies how to use A.I. will be a big business.” ”
Ask the average passerby on the street to describe artificial intelligence and you’re apt to get answers like C-3PO and Apple’s Siri. But for those who follow AI developments on a regular basis and swim just below the surface of the broad field , the idea that the foreseeable AI future might be driven more by Big Data rather than big discoveries is probably not a huge surprise. In a recent interview with Data Scientist and Entrepreneur Eyal Amir, we discussed how companies are using AI to connect the dots between data and innovation.
Image credit: Startup Leadership Program ChicagoAccording to Amir, the ability to make connections between big data together has quietly become a strong force in a number of industries. In advertising for example, companies can now tease apart data to discern the basics of who you are, what you’re doing, and where you’re going, and tailor ads to you based on that information.
“What we need to understand is that, most of the time, the data is not actually available out there in the way we think that it is. So, for example I don’t know if a user is a man or woman. I don’t know what amounts of money she’s making every year. I don’t know where she’s working,” said Eyal. “There are a bunch of pieces of data out there, but they are all suggestive. (But) we can connect the dots and say, ‘she’s likely working in banking based on her contacts and friends.’ It’s big machines that are crunching this.”
Amir used the example of image recognition to illustrate how AI is connecting the dots to make inferences and facilitate commerce. Many computer programs can now detect the image of a man on a horse in a photograph. Yet many of them miss the fact that, rather than an actual man on a horse, the image is actually a statue of a man on a horse. This lack of precision in analysis of broad data is part of what’s keep autonomous cars on the curb until the use of AI in commerce advances.
“You can connect the dots enough that you can create new applications, such as knowing where there is a parking spot available in the street. It doesn’t make financial sense to put sensors everywhere, so making those connections between a bunch of data sources leads to precise enough information that people are actually able to use,” Amir said. “Think about, ‘How long is the line at my coffee place down the street right now?’ or ‘Does this store have the shirt that I’m looking for?’ The information is not out there, but most companies don’t have a lot of incentive to put it out there for third parties. But there will be the ability to…infer a lot of that information.”
This greater ability to connect information and deliver more precise information through applications will come when everybody chooses to pool their information, said Eyal. While he expects a fair bit of resistance to that concept, Amir predicts that there will ultimately be enough players working together to infer and share information; this approach may provide more benefits on an aggregate level, as compared to an individual company that might not have the same incentives to share.
As more data is collected and analyzed, another trend that Eyal sees on the horizon is more autonomy being given to computers. Far from the dire predictions of runaway computers ruling the world, he sees a ‘supervised’ autonomy in which computers have the ability to perform tasks using knowledge that is out-of-reach for humans. Of course, this means developing a sense trust and allowing the computer to make more choices for us.
“The same way that we would let our TiVo record things that are of interest to us, it would still record what we want, but maybe it would record some extras. The same goes with (re-stocking) my groceries every week,” he said. “There is this trend of ‘Internet of Things,’ which brings together information about the contents of your refrigerator, for example. Then your favorite grocery store would deliver what you need without you having to spend an extra hour (shopping) every week.”
On the other hand, Amir does have some potential concerns about the future of artificial intelligence, comparable to what’s been voiced by Elon Musk and others. Yet he emphasizes that it’s not just the technology we should be concerned about.
“At the end, this will be AI controlled by market forces. I think the real risk is not the technology, but the combination of technology and market forces. That, together, poses some threats,” Amir said. “I don’t think that the computers themselves, in the foreseeable future, will terminate us because they want to. But they may terminate us because the hackers wanted to.”
“Apple Inc. has purchased Emotient Inc., a startup that uses artificial-intelligence technology to read people’s emotions by analyzing facial expressions.”
“When the world’s smartest researchers train computers to become smarter, they like to use games. Go, the two-player board game born in China more than two millennia ago, remains the nut that machines still can’t crack.”
In spite of the popular perception of the state of artificial intelligence, technology has yet to create a robot with the same instincts and adaptability as a human. While humans are born with some natural instincts that have evolved over millions of years, Neuroscientist and Artificial Intelligence Expert Dr. Danko Nikolic believes these same tendencies can be instilled in a robot.
“Our biological children are born with a set of knowledge. They know where to learn, they know where to pay attention. Robots simply can not do that,” Nikolic said. “The problem is you can not program it. There’s a trick we can use called AI Kindergarten. Then we can basically interact with this robot kind of like we do with children in kindergarten, but then make robots learn one level lower, at the level of something called machine genome.”
Programming that machine genome would require all of the innate human knowledge that’s evolved over thousands of years, Nikolic said. Lacking that ability, he said researchers are starting from scratch. While this form of artificial intelligence is still in its embryonic state, it does have some evolutionary advantages that humans didn’t have.
“By using AI Kindergarten, we don’t have to repeat the evolution exactly the way evolution has done it,” Nikolic said. “This experiment has been done already and the knowledge is already stored in our genes, so we can accelerate tremendously. We can skip millions of failed experiments where evolution has failed already.”
Rather than jumping into logic or facial recognition, researchers must still begin with simple things, like basic reflexes and build on top of that, Nikolic said. From there, we can only hope to come close to the intelligence of an insect or small bird.
“I think we can develop robots that would be very much biological, like robots, and they would behave as some kind of lower level intelligence animal, like a cockroach or lesser intelligent birds,” he said. “(The robots) would behave the way (animals) do and they would solve problems the way they do. It would have the flexibility and adaptability that they have and that’s much, much more than what we have today.”
As that machine genome continues to evolve, Nikolic compared the potential manipulation of that genome to the selective breeding that ultimately evolved ferocious wolves into friendly dogs. The results of robotic evolution will be equally benign, and he believes, any attempts to develop so-called “killer robots” won’t happen overnight. Just as it takes roughly 20 years for a child to fully develop into an adult, Nikolic sees an equally long process for artificial intelligence to evolve.
Nikolic cited similar attempts in the past where the manipulation of the genome of biological systems produced a very benign result. Further, he doesn’t foresee researchers creating something dangerous, and given his theory that AI could develops from a core genome, then it would be next to impossible to change the genome of a machine or of a biological system by just changing a few parts.
Going forward, Nikolic still sees a need for caution. Building some form of malevolent artificial intelligence is possible, he said, but the degree of difficulty still makes it unlikely.
“We can not change the genome of machine or human simply by changing a few parts and then having the thing work as we want. Making it mean is much more difficult than developing a nuclear weapon,” Nikolic said. “I think we have things to watch out for, and there should be regulation, but I don’t think this is a place for some major fear… there is no big risk. What we will end up with, I believe, will be a very friendly AI that will care for humans and serve humans and that’s all we will ever use.”