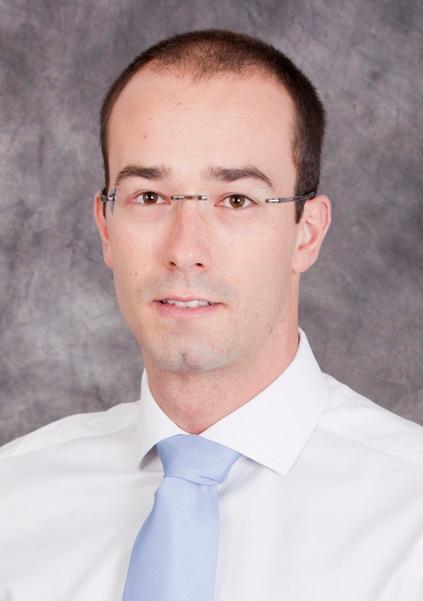
When you think of the words “data” and “mine”, no doubt the idea of data mining comes first. However, just as much as we find value in mining the rich resources of data, so too can we apply the advanced techniques for dealing with data to real-world mining — that is, extracting natural resources from the earth. The world is just as dependent on natural resources as it is data resources, so it makes sense to see how the evolving areas of artificial intelligence and machine learning have an impact on the world of mining and natural resource extraction.
Mining has always been a dangerous profession, since extracting minerals, natural gas, petroleum, and other resources requires working in conditions that can be dangerous for human life. Increasingly, we are needing to go to harsher climates such as deep under the ocean or deep inside the earth to extract the resources we still need. It should come as little surprise then that mining and resource extraction companies are looking to robotics, autonomous systems, and AI applications of all sorts to minimize risk, maximize return, and also lessen the environmental impact that mining has on our ecosystem.
On a recent AI Today podcast episode, Antoine Desmet of mining technology and equipment company Komatsu shared how they’re using advanced forms of AI, automation, and robotics to make an impact on the organization’s operations. Antoine has an interesting background, starting his career as a telecom engineer and receiving a Ph.D in neural network engineering. After getting his Ph.D, he returned to Komatsu and started working in surface analytics. He states that at the time there was a lot of data to work with, but very few analytics in place. He decided to start implementing machine learning and in the last few years his company has seen significant growth through this approach, with his data science team growing from just one person to ten people.