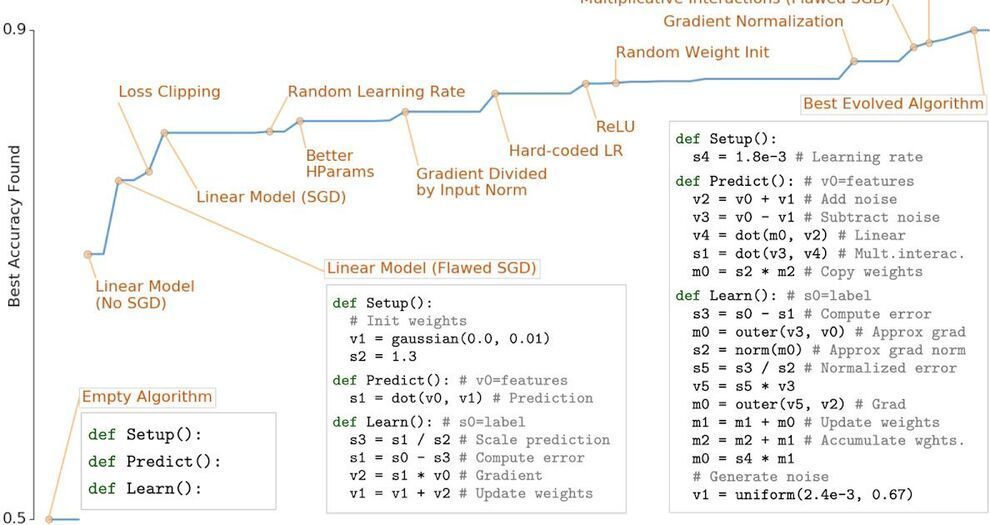
The snake bites its tail
Google AI can independently discover AI methods.
Then optimizes them
It Evolves algorithms from scratch—using only basic mathematical operations—rediscovering fundamental ML techniques & showing the potential to discover novel algorithms.
AutoML-Zero: new research that that can rediscover fundamental ML techniques by searching a space of different ways of combining basic mathematical operations. Arxiv: https://arxiv.org/abs/2003.
Machine learning (ML) has seen tremendous successes recently, which were made possible by ML algorithms like deep neural networks that were discovered through years of expert research. The difficulty involved in this research fueled AutoML, a field that aims to automate the design of ML algorithms. So far, AutoML has focused on constructing solutions by combining sophisticated hand-designed components. A typical example is that of neural architecture search, a subfield in which one builds neural networks automatically out of complex layers (e.g., convolutions, batch-norm, and dropout), and the topic of much research.
An alternative approach to using these hand-designed components in AutoML is to search for entire algorithms from scratch. This is challenging because it requires the exploration of vast and sparse search spaces, yet it has great potential benefits — it is not biased toward what we already know and potentially allows for the discovery of new and better ML architectures. By analogy, if one were building a house from scratch, there is more potential for flexibility or improvement than if one was constructing a house using only prefabricated rooms. However, the discovery of such housing designs may be more difficult because there are many more possible ways to combine the bricks and mortar than there are of combining pre-made designs of entire rooms. As such, early research into algorithm learning from scratch focused on one aspect of the algorithm, to reduce the search space and compute required, such as the learning rule, and has not been revisited much since the early 90s. Until now.
Extending our research into evolutionary AutoML, our recent paper, to be published at ICML 2020, demonstrates that it is possible to successfully evolve ML algorithms from scratch. The approach we propose, called AutoML-Zero, starts from empty programs and, using only basic mathematical operations as building blocks, applies evolutionary methods to automatically find the code for complete ML algorithms. Given small image classification problems, our method rediscovered fundamental ML techniques, such as 2-layer neural networks with backpropagation, linear regression and the like, which have been invented by researchers throughout the years. This result demonstrates the plausibility of automatically discovering more novel ML algorithms to address harder problems in the future.